Nic Encina
Nic is Director of Strategy of the Precision Population Health initiative at Ariadne Labs and oversees the research, data analytics, and technical teams. He is a cross-discipline specialist who has played key roles in building teams and pioneering innovation at technology and pharmaceutical companies.
Most recently, at the discretion of PerkinElmer’s CEO, Nic drove a new approach to innovation by establishing a new, semi-secret innovation lab and by leading the cloud and IOT strategies. Prior to that, He was CEO and founder of Wingu, which was one of Google’s first venture investments in health technology (acquired by PerkinElmer in 2013). He also played early leadership and technical roles at Infinity Pharmaceuticals and TransForm pharmaceuticals. Nic began his career in the Human Genome Project as an engineer in the B.L.A.S.T. lab of Warren Gish.
Nic is passionate about product innovation and market disruption as they pertain to real world problems in health & science. He is comfortable operating in environments where uncertainty is common, and particularly where the science of running an organization intersects with the art of harnessing value from teams to solve multi-disciplinary problems.
Nic earned BS degrees in biology and psychology from the University of Rochester, an MS in Cell Biology, an MS in Computer Science, and an MBA in healthcare from Yale School of Management.
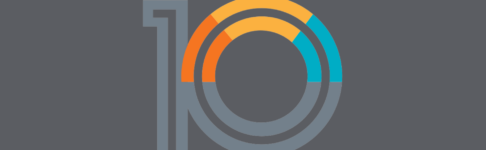
In our Five-Question series, we highlight the staff and faculty behind the compelling work at Ariadne Labs. Upstream or downstream? That has long been a career question for Nic Encina, MS, MS, MBA, now Director of Strategy of the Precision Population Health initiative at Ariadne Labs. In the medical and health world “upstream” is often… Continue reading Five Questions with Nic Encina: Working Upstream or Downstream
The International Consortium of Newborn Sequencing (ICoNS) published their findings and tool in Genetics in Medicine. They identified key factors influencing gene inclusion in newborn genomic screening programs and the team developed a machine learning model incorporating 13 predictors, achieving high accuracy in predicting gene selection across programs.
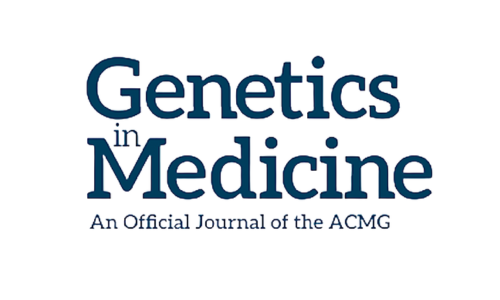